
Fairness in AI is the hardest question out of all AI socio-technical challenges because we are unable to define fairness. Political science has long been trying to answer this question which can also be applied to algorithmic fairness but it will never be sufficient. Attempts to define and apply fairness to algorithms should be interactive and progressive: it is a moving target which changes across locations, times and societies.
In algorithmic decision-making there are two major concerns about unfair outcomes which harms not only society at large but also businesses:
Amplifying existing human bias: algorithms learn from the data we feed them. That is the data which contains all biased human decision-making... because is there an unbiased human decision-making?
Generating new types of bias: algorithms so far cannot reason with causal relationships, they only find correlations in data. Those correlations might be flawed, skewd and simply wrong. But there is already ongoing research on Causal AI.
Why fair algorithmic decision-making is in fact beneficial for the business
Apple’s credit card algorithm gave Steve Wozniak 10 times more credit score than his wife even though they share all the same accounts and assets. Not very smart of the business, right? Or hiring algorithms filtering out possibly much more talented female candidate in exchange for less relevant male one. Moreover, a Boston Consulting Group study found that companies with more diverse teams have 19% higher revenues due to innovation and creativity.
Where is the solution
Most of the organisations that are setting the standards today are still elitists and homogeneous, voicing those countries, companies, entities or individuals that already have pretty high pitch voices. While they discuss inclusion, fairness, diversity, non-discrimination and decolonization the doors are closed for others, for exactly those discriminated, colonized or excluded.
First, ML developers, data scientists or computer scientists will not be able to resolve the problem of fairness alone, the problem is socio-technical.
Second, Fairness does not come from a top-down approach only, rather mostly bottom-up - while discussing inclusion, the discussion table must be inclusive! Empowering each and every voice makes a difference if we really want that difference. But those who really want and need that difference are not welcome to the decision-making table. The rest in many cases are negligent, those, privileged ones. Algorithmic fairness must be practiced progressively, inclusively, interactively and fairly with feedback loops.
Third, we must leverage technologies themselves to solve deeply rooted problems of unfairness. One important portion of the solution can be found in the tweet exchange of myself and Jack Clark of the AI Index.
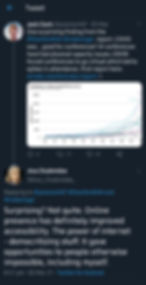
Right now we have an unprecedented opportunity to make a difference. We must build robust and reliable tools, frameworks, infrastructures and inclusive socio-technical systems. We have everything except enough will. The internet has no borders, internet needs no visas, internet has no doors, internet has no social strata. All technologies have the capability to lift all the restrictions from those restricted.
Let it happen. Make it happen.
Jack’s response by the way.
